**The podcast is currently on hiatus. For more active NLP content, check out the Holistic Intelligence Podcast linked below.** Welcome to the NLP highlights podcast, where we invite researchers to talk about their work in various areas in natural language processing. All views expressed belong to the hosts/guests, and do not represent their employers.
…
continue reading
Curious about the safety of LLMs? 🤔 Join us for an insightful new episode featuring Suchin Gururangan, Young Investigator at Allen Institute for Artificial Intelligence and Data Science Engineer at Appuri. 🚀 Don't miss out on expert insights into the world of LLMs!Di Allen Institute for Artificial Intelligence
…
continue reading
This podcast episode features Dr. Mohamed Elhoseiny, a true luminary in the realm of computer vision with over a decade of groundbreaking research. As an Assistant Professor at KAUST, Dr. Elhoseiny's work delves into the intersections of Computer Vision, Language & Vision, and Computational Creativity in Art, Fashion, and AI. Notably, he co-organiz…
…
continue reading
Our first guest with this new format is Kyle Lo, the most senior lead scientist in the Semantic Scholar team at Allen Institute for AI (AI2), who kindly agreed to share his perspective on #Science of #Science (#scisci) on our podcast. SciSci is concerned with studying how people do science, and includes developing methods and tools to help people c…
…
continue reading
In this special episode of NLP Highlights, we discussed building and open sourcing language models. What is the usual recipe for building large language models? What does it mean to open source them? What new research questions can we answer by open sourcing them? We particularly focused on the ongoing Open Language Model (OLMo) project at AI2, and…
…
continue reading
In this special episode, we chatted with Chris Callison-Burch about his testimony in the recent U.S. Congress Hearing on the Interoperability of AI and Copyright Law. We started by asking Chris about the purpose and the structure of this hearing. Then we talked about the ongoing discussion on how the copyright law is applicable to content generated…
…
continue reading
How can we generate coherent long stories from language models? Ensuring that the generated story has long range consistency and that it conforms to a high level plan is typically challenging. In this episode, Kevin Yang describes their system that prompts language models to first generate an outline, and iteratively generate the story while follow…
…
continue reading
Compositional generalization refers to the capability of models to generalize to out-of-distribution instances by composing information obtained from the training data. In this episode we chatted with Najoung Kim, on how to explicitly evaluate specific kinds of compositional generalization in neural network models of language. Najoung described COG…
…
continue reading
We invited Urvashi Khandelwal, a research scientist at Google Brain to talk about nearest neighbor language and machine translation models. These models interpolate parametric (conditional) language models with non-parametric distributions over the closest values in some data stores built from relevant data. Not only are these models shown to outpe…
…
continue reading
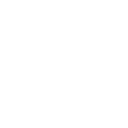
1
136 - Including Signed Languages in NLP, with Kayo Yin and Malihe Alikhani
1:02:15
1:02:15
Riproduci in seguito
Riproduci in seguito
Liste
Like
Like aggiunto
1:02:15
In this episode, we talk with Kayo Yin, an incoming PhD at Berkeley, and Malihe Alikhani, an assistant professor at the University of Pittsburgh, about opportunities for the NLP community to contribute to Sign Language Processing (SLP). We talked about history and misconceptions about sign languages, high-level similarities and differences between …
…
continue reading
This episode is the third in our current series on PhD applications.We talk about what the PhD application process looks like after applications are submitted. We start with a general overview of the timeline, then talk about how to approach interviews and conversations with faculty, and finish by discussing the different factors to consider in dec…
…
continue reading
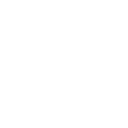
1
134 - PhD Application Series: PhDs in Europe versus the US, with Barbara Plank and Gonçalo Correia
38:29
This episode is the second in our current series on PhD applications.How do PhD programs in Europe differ from PhD programs in the US, and how should people decide between them? In this episode, we invite Barbara Plank (Professor at ITU, IT University of Copenhagen) and Gonçalo Correia (ELLIS PhD student at University of Lisbon and University of Am…
…
continue reading
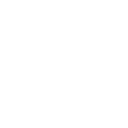
1
133 - PhD Application Series: Preparing Application Materials, with Nathan Schneider and Roma Patel
43:54
This episode is the first in our current series on PhD applications.How should people prepare their applications to PhD programs in NLP? In this episode, we invite Nathan Schneider (Professor of Linguistics and Computer Science at Georgetown University) and Roma Patel (PhD student in Computer Science at Brown University) to share their perspectives…
…
continue reading
In this episode, we discussed the Alexa Prize Socialbot Grand Challenge and this year's winning submission, Alquist 4.0, with Petr Marek, a member of the winning team. Petr gave us an overview of their submission, the design choices that led to them winning the competition, including combining a hardcoded dialog tree and a neural generator model an…
…
continue reading
What can NLP researchers learn from Human Computer Interaction (HCI) research? We chatted with Nanna Inie and Leon Derczynski to find out. We discussed HCI's research processes including methods of inquiry, the data annotation processes used in HCI, and how they are different from NLP, and the cognitive methods used in HCI for qualitative error ana…
…
continue reading
In this episode, we talk with Lisa Beinborn, an assistant professor at Vrije Universiteit Amsterdam, about how to use human cognitive signals to improve and analyze NLP models. We start by discussing different kinds of cognitive signals—eye-tracking, EEG, MEG, and fMRI—and challenges associated with using them. We then turn to Lisa’s recent work co…
…
continue reading
In this episode, we talk to Shunyu Yao about recent insights into how transformers can represent hierarchical structure in language. Bounded-depth hierarchical structure is thought to be a key feature of natural languages, motivating Shunyu and his coauthors to show that transformers can efficiently represent bounded-depth Dyck languages, which can…
…
continue reading
We discussed adversarial dataset construction and dynamic benchmarking in this episode with Douwe Kiela, a research scientist at Facebook AI Research who has been working on a dynamic benchmarking platform called Dynabench. Dynamic benchmarking tries to address the issue of many recent datasets getting solved with little progress being made towards…
…
continue reading
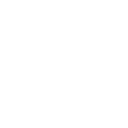
1
127 - Masakhane and Participatory Research for African Languages, with Tosin Adewumi and Perez Ogayo
47:17
We invited members of Masakhane, Tosin Adewumi and Perez Ogayo, to talk about their EMNLP Findings paper that discusses why typical research is limited for low-resourced NLP and how participatory research can help. As a result of participatory research, Masakhane has many, many success stories: first datasets and benchmarks in African languages, fi…
…
continue reading
We invited Lisa Li to talk about her recent work, Prefix-Tuning: Optimizing Continuous Prompts for Generation. Prefix tuning is a lightweight alternative to finetuning, and the idea is to tune only a fixed-length task-specific continuous vector, and to keep the pretrained transformer parameters frozen. We discussed how prefix tuning compares with f…
…
continue reading
How can we build Visual Question Answering systems for real users? For this episode, we chatted with Danna Gurari, about her work in building datasets and models towards VQA for people who are blind. We talked about the differences between the existing datasets, and Vizwiz, a dataset built by Gurari et al., and the resulting algorithmic changes. We…
…
continue reading
We invited Jayant Krishnamurthy and Hao Fang, researchers at Microsoft Semantic Machines to discuss their platform for building task-oriented dialog systems, and their recent TACL paper on the topic. The paper introduces a new formalism for task-oriented dialog to effectively handle references and revisions in complex dialog, and a large realistic …
…
continue reading
In this episode, Robin Jia talks about how to build robust NLP systems. We discuss the different senses in which a system can be robust, reasons to care about system robustness, and the challenges involved in evaluating robustness of NLP models. We talk about how to build certifiably robust models through interval bound propagation and discrete enc…
…
continue reading
We invited Nils Holzenberger, a PhD student at JHU to talk about a dataset involving statutory reasoning in tax law Holzenberger et al. released recently. This dataset includes difficult textual entailment and question answering problems that involve reasoning about how sections in tax law are applicable to specific cases. They also released a Prol…
…
continue reading
We invited Alona Fyshe to talk about the link between NLP and the human brain. We began by talking about what we currently know about the connection between representations used in NLP and representations recorded in the brain. We also discussed how different brain imaging techniques compare to each other. We then dove into experiments investigatin…
…
continue reading
We invited Asli Celikyilmaz for this episode to talk about evaluation of text generation systems. We discussed the challenges in evaluating generated text, and covered human and automated metrics, with a discussion of recent developments in learning metrics. We also talked about some open research questions, including the difficulties in evaluating…
…
continue reading
In this episode, Diyi Yang gives us an overview of using NLP models for social applications, including understanding social relationships, processes, roles, and power. As NLP systems are getting used more and more in the real world, they additionally have increasing social impacts that must be studied. We talk about how to get started in this field…
…
continue reading
In this episode, we talked about Coreference Resolution with Marta Recasens, a Research Scientist at Google. We discussed the complexity involved in resolving references in language, the simplification of the problem that the NLP community has focused on by talking about specific datasets, and the complex coreference phenomena that are not yet capt…
…
continue reading
We interviewed Sameer Singh for this episode, and discussed an overview of recent work in interpreting NLP model predictions, particularly instance-level interpretations. We started out by talking about why it is important to interpret model outputs and why it is a hard problem. We then dove into the details of three kinds of interpretation techniq…
…
continue reading
We invited Yonatan Bisk to talk about grounded language understanding. We started off by discussing an overview of the topic, its research goals, and the the challenges involved. In the latter half of the conversation, we talked about ALFRED (Shridhar et al., 2019), a grounded instruction following benchmark that simulates training a robot butler. …
…
continue reading
In this special episode, Carissa Schoenick, a program manager and communications director at AI2 interviewed Matt Gardner about AllenNLP. We chatted about the origins of AllenNLP, the early challenges in building it, and the design decisions behind the library. Given the release of AllenNLP 1.0 this week, we asked Matt what users can expect from th…
…
continue reading
We invited Marco Tulio Ribeiro, a Senior Researcher at Microsoft, to talk about evaluating NLP models using behavioral testing, a framework borrowed from Software Engineering. Marco describes three kinds of black-box tests the check whether NLP models satisfy certain necessary conditions. While breaking the standard IID assumption, this framework p…
…
continue reading
We invited Fernando Pereira, a VP and Distinguished Engineer at Google, where he leads NLU and ML research, to talk about managing NLP research teams in industry. Topics we discussed include prioritizing research against product development and effective collaboration with product teams, dealing with potential research interest mismatch between ind…
…
continue reading
We invited Steven Cao to talk about his paper on multilingual alignment of contextual word embeddings. We started by discussing how multilingual transformers work in general, and then focus on Steven’s work on aligning word representations. The core idea is to start from a list of words automatically aligned from parallel corpora and to ensure the …
…
continue reading
We invited Jon Clark from Google to talk about TyDi QA, a new question answering dataset, for this episode. The dataset contains information seeking questions in 11 languages that are typologically diverse, i.e., they differ from each other in terms of key structural and functional features. The questions in TyDiQA are information-seeking, like tho…
…
continue reading
In this episode, Tom Kwiatkowski and Michael Collins talk about Natural Questions, a benchmark for question answering research. We discuss how the dataset was collected to reflect naturally-occurring questions, the criteria used for identifying short and long answers, how this dataset differs from other QA datasets, and how easy it might be to game…
…
continue reading
How do we know, in a concrete quantitative sense, what a deep learning model knows about language? In this episode, Ellie Pavlick talks about two broad directions to address this question: structural and behavioral analysis of models. In structural analysis, we often train a linear classifier for some linguistic phenomenon we'd like to probe (e.g.,…
…
continue reading
In this episode we invite Verena Rieser and Ondřej Dušek on to talk to us about the complexities of generating natural language when you have some kind of structured meaning representation as input. We talk about when you might want to do this, which is often is some kind of a dialog system, but also generating game summaries, and even some langu…
…
continue reading
In this episode, we invite Hao Tan and Mohit Bansal to talk about multi-modal training of transformers, focusing in particular on their EMNLP 2019 paper that introduced LXMERT, a vision+language transformer. We spend the first third of the episode talking about why you might want to have multi-modal representations. We then move to the specifics of…
…
continue reading
In this episode, we talked to Emily Bender about the ethical considerations in developing NLP models and putting them in production. Emily cited specific examples of ethical issues, and talked about the kinds of potential concerns to keep in mind, both when releasing NLP models that will be used by real people, and also while conducting NLP researc…
…
continue reading
In this episode we invite Sudha Rao to talk about question generation. We talk about different settings where you might want to generate questions: for human testing scenarios (rare), for data augmentation (has been done a bunch for SQuAD-like tasks), for detecting missing information / asking clarification questions, for dialog uses, and others. A…
…
continue reading
In this episode we talked with Victor Sanh and Thomas Wolf from HuggingFace about model distillation, and DistilBERT as one example of distillation. The idea behind model distillation is compressing a large model by building a smaller model, with much fewer parameters, that approximates the output distribution of the original model, typically for i…
…
continue reading
We talked to Brendan O’Connor for this episode about processing language in social media. Brendan started off by telling us about his projects that studied the linguistic and geographical patterns of African American English (AAE), and how obtaining data from Twitter made these projects possible. We then talked about how many tools built for standa…
…
continue reading
What exciting NLP research problems are involved in processing biomedical and clinical data? In this episode, we spoke with Dina Demner-Fushman, who leads NLP and IR research at the Lister Hill National Center for Biomedical Communications, part of the National Library of Medicine. We talked about processing biomedical scientific literature, unders…
…
continue reading
In this episode, Jonathan Frankle describes the lottery ticket hypothesis, a popular explanation of how over-parameterization helps in training neural networks. We discuss pruning methods used to uncover subnetworks (winning tickets) which were initialized in a particularly effective way. We also discuss patterns observed in pruned networks, stabil…
…
continue reading
For our 100th episode, we invite AI2 CEO Oren Etzioni to talk to us about NLP startups. Oren has founded several successful startups, is himself an investor in startups, and helps with AI2's startup incubator.Some of our discussion topics include: What's the similarity between being a researcher and an entrepreneur? How do you transition from being…
…
continue reading
For this episode, we chatted with Neil Thomas and Roshan Rao about modeling protein sequences and evaluating transfer learning methods for a set of five protein modeling tasks. Learning representations using self-supervised pretaining objectives has shown promising results in transferring to downstream tasks in protein sequence modeling, just like …
…
continue reading
What function do the different attention heads serve in multi-headed attention models? In this episode, Lena describes how to use attribution methods to assess the importance and contribution of different heads in several tasks, and describes a gating mechanism to prune the number of effective heads used when combined with an auxiliary loss. Then, …
…
continue reading
In this episode, we talk to Taylor Berg-Kirkpatrick about optical character recognition (OCR) on historical documents. Taylor starts off by describing some practical issues related to old scanning processes of documents that make performing OCR on them a difficult problem. Then he explains how one can build latent variable models for this data usin…
…
continue reading
In this episode, we chat with Luke Zettlemoyer about Question Answering as a format for crowdsourcing annotations of various semantic phenomena in text. We start by talking about QA-SRL and QAMR, two datasets that use QA pairs to annotate predicate-argument relations at the sentence level. Luke describes how this annotation scheme makes it possible…
…
continue reading
In this episode, we invite Yejin Choi to talk about common sense knowledge and reasoning, a growing area in NLP. We start by discussing a working definition of “common sense” and the practical utility of studying it. We then talk about some of the datasets and resources focused on studying different aspects of common sense (e.g., ReCoRD, Commonsens…
…
continue reading