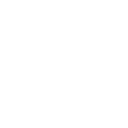
Vai offline con l'app Player FM !
Boost Your Model: Wie funktioniert Data-Science im Maschinenraum?
Manage episode 452217722 series 3394916
Summary: In this conversation, Aleksander Fegel and Dr. David Geisel discuss the critical aspects of feature selection and engineering in data science. They explore the importance of understanding features, the role of data quality, and the necessity of engaging with domain experts. The discussion covers various methods of feature selection, including filter methods, wrapper methods, and embedded methods, emphasizing the need for simplicity and clarity in model building. The conversation concludes with insights on how to effectively interpret model predictions and the significance of collaboration in data science projects.
Takeaways: -Feature selection is essential for successful data modeling. -Data quality significantly impacts model performance. -Engaging with domain experts enhances feature selection. -Simplicity in models often leads to better results. -Feature importance helps in understanding model predictions. -Different methods exist for feature selection, each with pros and cons. -Collaboration between data scientists and domain experts is crucial. -Feature engineering is a continuous process in data science. -Understanding the context of data is vital for effective modeling. -Iterative testing and validation are key to successful feature selection. titles
19 episodi
Manage episode 452217722 series 3394916
Summary: In this conversation, Aleksander Fegel and Dr. David Geisel discuss the critical aspects of feature selection and engineering in data science. They explore the importance of understanding features, the role of data quality, and the necessity of engaging with domain experts. The discussion covers various methods of feature selection, including filter methods, wrapper methods, and embedded methods, emphasizing the need for simplicity and clarity in model building. The conversation concludes with insights on how to effectively interpret model predictions and the significance of collaboration in data science projects.
Takeaways: -Feature selection is essential for successful data modeling. -Data quality significantly impacts model performance. -Engaging with domain experts enhances feature selection. -Simplicity in models often leads to better results. -Feature importance helps in understanding model predictions. -Different methods exist for feature selection, each with pros and cons. -Collaboration between data scientists and domain experts is crucial. -Feature engineering is a continuous process in data science. -Understanding the context of data is vital for effective modeling. -Iterative testing and validation are key to successful feature selection. titles
19 episodi
Tutti gli episodi
×Benvenuto su Player FM!
Player FM ricerca sul web podcast di alta qualità che tu possa goderti adesso. È la migliore app di podcast e funziona su Android, iPhone e web. Registrati per sincronizzare le iscrizioni su tutti i tuoi dispositivi.