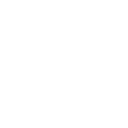
Vai offline con l'app Player FM !
Why Your GPUs are underutilised for AI - CentML CEO Explains
Manage episode 450014752 series 2803422
Prof. Gennady Pekhimenko (CEO of CentML, UofT) joins us in this *sponsored episode* to dive deep into AI system optimization and enterprise implementation. From NVIDIA's technical leadership model to the rise of open-source AI, Pekhimenko shares insights on bridging the gap between academic research and industrial applications. Learn about "dark silicon," GPU utilization challenges in ML workloads, and how modern enterprises can optimize their AI infrastructure. The conversation explores why some companies achieve only 10% GPU efficiency and practical solutions for improving AI system performance. A must-watch for anyone interested in the technical foundations of enterprise AI and hardware optimization.
CentML offers competitive pricing for GenAI model deployment, with flexible options to suit a wide range of models, from small to large-scale deployments. Cheaper, faster, no commitments, pay as you go, scale massively, simple to setup. Check it out!
https://centml.ai/pricing/
SPONSOR MESSAGES:
MLST is also sponsored by Tufa AI Labs - https://tufalabs.ai/
They are hiring cracked ML engineers/researchers to work on ARC and build AGI!
SHOWNOTES (diarised transcript, TOC, references, summary, best quotes etc)
https://www.dropbox.com/scl/fi/w9kbpso7fawtm286kkp6j/Gennady.pdf?rlkey=aqjqmncx3kjnatk2il1gbgknk&st=2a9mccj8&dl=0
TOC:
1. AI Strategy and Leadership
[00:00:00] 1.1 Technical Leadership and Corporate Structure
[00:09:55] 1.2 Open Source vs Proprietary AI Models
[00:16:04] 1.3 Hardware and System Architecture Challenges
[00:23:37] 1.4 Enterprise AI Implementation and Optimization
[00:35:30] 1.5 AI Reasoning Capabilities and Limitations
2. AI System Development
[00:38:45] 2.1 Computational and Cognitive Limitations of AI Systems
[00:42:40] 2.2 Human-LLM Communication Adaptation and Patterns
[00:46:18] 2.3 AI-Assisted Software Development Challenges
[00:47:55] 2.4 Future of Software Engineering Careers in AI Era
[00:49:49] 2.5 Enterprise AI Adoption Challenges and Implementation
3. ML Infrastructure Optimization
[00:54:41] 3.1 MLOps Evolution and Platform Centralization
[00:55:43] 3.2 Hardware Optimization and Performance Constraints
[01:05:24] 3.3 ML Compiler Optimization and Python Performance
[01:15:57] 3.4 Enterprise ML Deployment and Cloud Provider Partnerships
4. Distributed AI Architecture
[01:27:05] 4.1 Multi-Cloud ML Infrastructure and Optimization
[01:29:45] 4.2 AI Agent Systems and Production Readiness
[01:32:00] 4.3 RAG Implementation and Fine-Tuning Considerations
[01:33:45] 4.4 Distributed AI Systems Architecture and Ray Framework
5. AI Industry Standards and Research
[01:37:55] 5.1 Origins and Evolution of MLPerf Benchmarking
[01:43:15] 5.2 MLPerf Methodology and Industry Impact
[01:50:17] 5.3 Academic Research vs Industry Implementation in AI
[01:58:59] 5.4 AI Research History and Safety Concerns
216 episodi
Manage episode 450014752 series 2803422
Prof. Gennady Pekhimenko (CEO of CentML, UofT) joins us in this *sponsored episode* to dive deep into AI system optimization and enterprise implementation. From NVIDIA's technical leadership model to the rise of open-source AI, Pekhimenko shares insights on bridging the gap between academic research and industrial applications. Learn about "dark silicon," GPU utilization challenges in ML workloads, and how modern enterprises can optimize their AI infrastructure. The conversation explores why some companies achieve only 10% GPU efficiency and practical solutions for improving AI system performance. A must-watch for anyone interested in the technical foundations of enterprise AI and hardware optimization.
CentML offers competitive pricing for GenAI model deployment, with flexible options to suit a wide range of models, from small to large-scale deployments. Cheaper, faster, no commitments, pay as you go, scale massively, simple to setup. Check it out!
https://centml.ai/pricing/
SPONSOR MESSAGES:
MLST is also sponsored by Tufa AI Labs - https://tufalabs.ai/
They are hiring cracked ML engineers/researchers to work on ARC and build AGI!
SHOWNOTES (diarised transcript, TOC, references, summary, best quotes etc)
https://www.dropbox.com/scl/fi/w9kbpso7fawtm286kkp6j/Gennady.pdf?rlkey=aqjqmncx3kjnatk2il1gbgknk&st=2a9mccj8&dl=0
TOC:
1. AI Strategy and Leadership
[00:00:00] 1.1 Technical Leadership and Corporate Structure
[00:09:55] 1.2 Open Source vs Proprietary AI Models
[00:16:04] 1.3 Hardware and System Architecture Challenges
[00:23:37] 1.4 Enterprise AI Implementation and Optimization
[00:35:30] 1.5 AI Reasoning Capabilities and Limitations
2. AI System Development
[00:38:45] 2.1 Computational and Cognitive Limitations of AI Systems
[00:42:40] 2.2 Human-LLM Communication Adaptation and Patterns
[00:46:18] 2.3 AI-Assisted Software Development Challenges
[00:47:55] 2.4 Future of Software Engineering Careers in AI Era
[00:49:49] 2.5 Enterprise AI Adoption Challenges and Implementation
3. ML Infrastructure Optimization
[00:54:41] 3.1 MLOps Evolution and Platform Centralization
[00:55:43] 3.2 Hardware Optimization and Performance Constraints
[01:05:24] 3.3 ML Compiler Optimization and Python Performance
[01:15:57] 3.4 Enterprise ML Deployment and Cloud Provider Partnerships
4. Distributed AI Architecture
[01:27:05] 4.1 Multi-Cloud ML Infrastructure and Optimization
[01:29:45] 4.2 AI Agent Systems and Production Readiness
[01:32:00] 4.3 RAG Implementation and Fine-Tuning Considerations
[01:33:45] 4.4 Distributed AI Systems Architecture and Ray Framework
5. AI Industry Standards and Research
[01:37:55] 5.1 Origins and Evolution of MLPerf Benchmarking
[01:43:15] 5.2 MLPerf Methodology and Industry Impact
[01:50:17] 5.3 Academic Research vs Industry Implementation in AI
[01:58:59] 5.4 AI Research History and Safety Concerns
216 episodi
Wszystkie odcinki
×Benvenuto su Player FM!
Player FM ricerca sul web podcast di alta qualità che tu possa goderti adesso. È la migliore app di podcast e funziona su Android, iPhone e web. Registrati per sincronizzare le iscrizioni su tutti i tuoi dispositivi.