Looks like the publisher may have taken this series offline or changed its URL. Please contact support if you believe it should be working, the feed URL is invalid, or you have any other concerns about it.
Vai offline con l'app Player FM !
Challenges in Evaluating AI Systems
Fetch error
Hmmm there seems to be a problem fetching this series right now. Last successful fetch was on January 02, 2025 12:05 (
What now? This series will be checked again in the next hour. If you believe it should be working, please verify the publisher's feed link below is valid and includes actual episode links. You can contact support to request the feed be immediately fetched.
Manage episode 424744792 series 3498845
Most conversations around the societal impacts of artificial intelligence (AI) come down to discussing some quality of an AI system, such as its truthfulness, fairness, potential for misuse, and so on. We are able to talk about these characteristics because we can technically evaluate models for their performance in these areas. But what many people working inside and outside of AI don’t fully appreciate is how difficult it is to build robust and reliable model evaluations. Many of today’s existing evaluation suites are limited in their ability to serve as accurate indicators of model capabilities or safety.
At Anthropic, we spend a lot of time building evaluations to better understand our AI systems. We also use evaluations to improve our safety as an organization, as illustrated by our Responsible Scaling Policy. In doing so, we have grown to appreciate some of the ways in which developing and running evaluations can be challenging.
Here, we outline challenges that we have encountered while evaluating our own models to give readers a sense of what developing, implementing, and interpreting model evaluations looks like in practice.
Source:
https://www.anthropic.com/news/evaluating-ai-systems
Narrated for AI Safety Fundamentals by Perrin Walker
A podcast by BlueDot Impact.
Learn more on the AI Safety Fundamentals website.
Capitoli
1. Challenges in Evaluating AI Systems (00:00:00)
2. Introduction (00:00:15)
3. Challenges (00:02:23)
4. The supposedly simple multiple-choice evaluation (00:02:25)
5. One size doesn't fit all when it comes to third-party evaluation frameworks (00:06:42)
6. The subjectivity of human evaluations (00:10:45)
7. The ouroboros of model-generated evaluations (00:15:29)
8. Preserving the objectivity of third-party audits while leveraging internal expertise (00:16:56)
9. Policy recommendations (00:18:44)
10. Conclusion (00:21:50)
85 episodi
Fetch error
Hmmm there seems to be a problem fetching this series right now. Last successful fetch was on January 02, 2025 12:05 (
What now? This series will be checked again in the next hour. If you believe it should be working, please verify the publisher's feed link below is valid and includes actual episode links. You can contact support to request the feed be immediately fetched.
Manage episode 424744792 series 3498845
Most conversations around the societal impacts of artificial intelligence (AI) come down to discussing some quality of an AI system, such as its truthfulness, fairness, potential for misuse, and so on. We are able to talk about these characteristics because we can technically evaluate models for their performance in these areas. But what many people working inside and outside of AI don’t fully appreciate is how difficult it is to build robust and reliable model evaluations. Many of today’s existing evaluation suites are limited in their ability to serve as accurate indicators of model capabilities or safety.
At Anthropic, we spend a lot of time building evaluations to better understand our AI systems. We also use evaluations to improve our safety as an organization, as illustrated by our Responsible Scaling Policy. In doing so, we have grown to appreciate some of the ways in which developing and running evaluations can be challenging.
Here, we outline challenges that we have encountered while evaluating our own models to give readers a sense of what developing, implementing, and interpreting model evaluations looks like in practice.
Source:
https://www.anthropic.com/news/evaluating-ai-systems
Narrated for AI Safety Fundamentals by Perrin Walker
A podcast by BlueDot Impact.
Learn more on the AI Safety Fundamentals website.
Capitoli
1. Challenges in Evaluating AI Systems (00:00:00)
2. Introduction (00:00:15)
3. Challenges (00:02:23)
4. The supposedly simple multiple-choice evaluation (00:02:25)
5. One size doesn't fit all when it comes to third-party evaluation frameworks (00:06:42)
6. The subjectivity of human evaluations (00:10:45)
7. The ouroboros of model-generated evaluations (00:15:29)
8. Preserving the objectivity of third-party audits while leveraging internal expertise (00:16:56)
9. Policy recommendations (00:18:44)
10. Conclusion (00:21:50)
85 episodi
Tutti gli episodi
×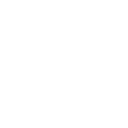
1 Constitutional AI Harmlessness from AI Feedback 1:01:49
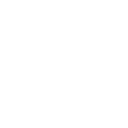
1 Intro to Brain-Like-AGI Safety 1:02:10
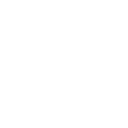
1 Eliciting Latent Knowledge 1:00:27
Benvenuto su Player FM!
Player FM ricerca sul web podcast di alta qualità che tu possa goderti adesso. È la migliore app di podcast e funziona su Android, iPhone e web. Registrati per sincronizzare le iscrizioni su tutti i tuoi dispositivi.